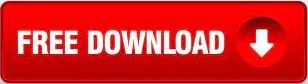

$ estimate nonmem important sampling software#
NONMEM software assisted by Pirana, PsN, and Xpose was used to estimate population PK parameters, and R program was used to analyze and plot the results. A case study was performed with a clinical data of theophylline available in NONMEM distribution media. Relative root mean squared error (rRMSE) and relative estimation error (REE) were used to analyze the differences between true and estimated values. A stochastic simulation and estimation (SSE) study was performed to simultaneously simulate data sets and estimate the parameters using four different methods: FOCE-I only, BAYES(C) (FOCE-I and BAYES composite method), BAYES(F) (BAYES with all true initial parameters and fixed ω 2), and BAYES only. In this study, 100 data sets were simulated with eight sampling points for each subject and with six different levels of IIV (5%, 10%, 20%, 30%, 50%, and 80%) in their PK parameter distribution. In this study, the performance of a classical first-order conditional estimation with interaction (FOCE-I) and expectation maximization (EM)-based Markov chain Monte Carlo Bayesian (BAYES) estimation methods were compared for estimating the population parameters and its distribution from data sets having a low number of subjects. Exploratory preclinical, as well as clinical trials, may involve a small number of patients, making it difficult to calculate and analyze the pharmacokinetic (PK) parameters, especially if the PK parameters show very high inter-individual variability (IIV).
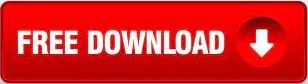